Lumos: Learning to Relight Portrait Images via a Virtual Light Stage and Synthetic-to-Real Adaptation
†NVIDIA Corporation
‡UC San Diego
SIGGRAPH Asia 2022
TL;DR: A relighting framework trained on paired synthetic data and adapted on unpaired real data.
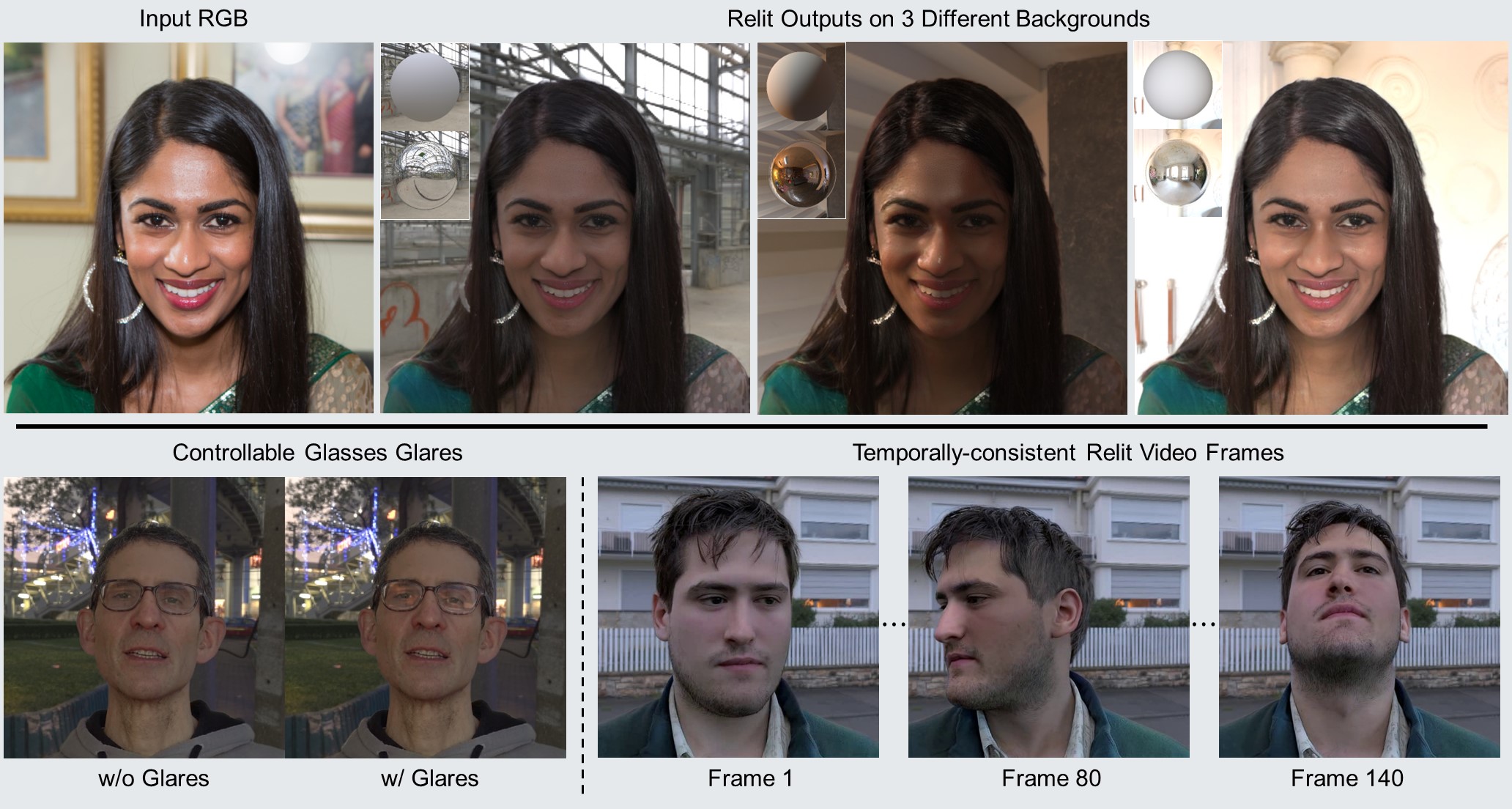
Abstract: Given a portrait image of a person and an environment map of the target lighting, portrait relighting aims to re-illuminate the person in the image as if the person appeared in an environment with the target lighting. To achieve high-quality results, recent methods rely on deep learning. An effective approach is to supervise the training of deep neural networks with a high-fidelity dataset of desired input-output pairs, captured with a light stage. However, acquiring such data requires an expensive special capture rig and time-consuming efforts, limiting access to only a few resourceful laboratories. To address the limitation, we propose a new approach that can perform on par with the state-of-the-art (SOTA) relighting methods without requiring a light stage. In addition to achieving SOTA results, our approach offers several advantages over the prior methods, including controllable glares on glasses and more temporally-consistent results for relighting videos.