A 0.32–128 TOPS, Scalable Multi-Chip-Module-Based Deep Neural Network Inference Accelerator With Ground-Referenced Signaling in 16 nm
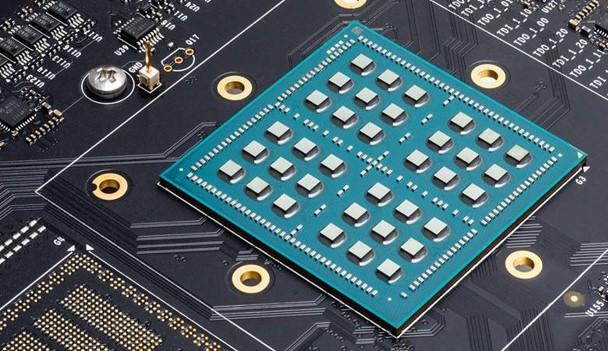
Custom accelerators improve the energy efficiency, area efficiency, and performance of deep neural network (DNN) inference. This article presents a scalable DNN accelerator consisting of 36 chips connected in a mesh network on a multi-chip-module (MCM) using ground-referenced signaling (GRS). While previous accelerators fabricated on a single monolithic chip are optimal for specific network sizes, the proposed architecture enables flexible scaling for efficient inference on a wide range of DNNs, from mobile to data center domains. Communication energy is minimized with large on-chip distributed weight storage and a hierarchical network-on-chip and network-on-package, and inference energy is minimized through extensive data reuse. The 16-nm prototype achieves 1.29-TOPS/mm^2 area efficiency, 0.11 pJ/op (9.5 TOPS/W) energy efficiency, 4.01-TOPS peak performance for a one-chip system, and 127.8 peak TOPS and 1903 images/s ResNet-50 batch-1 inference for a 36-chip system.
Publication Date
Published in
Award
Copyright
This material is posted here with permission of the IEEE. Internal or personal use of this material is permitted. However, permission to reprint/republish this material for advertising or promotional purposes or for creating new collective works for resale or redistribution must be obtained from the IEEE by writing to pubs-permissions@ieee.org.