SPGISpeech: 5,000 Hours of Transcribed Financial Audio for Fully Formatted End-to-End Speech Recognition
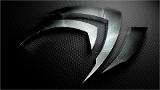
In the English speech-to-text (STT) machine learning task, acoustic models are conventionally trained on uncased Latin characters, and any necessary orthography (such as capitalization, punctuation, and denormalization of non-standard words) is imputed by separate post-processing models. This adds complexity and limits performance, as many formatting tasks benefit from semantic information present in the acoustic signal but absent in transcription. Here we propose a new STT task: end-to-end neural transcription with fully formatted text for target labels. We present baseline Conformer-based models trained on a corpus of 5,000 hours of professionally transcribed earnings calls, achieving a CER of 1.7. As a contribution to the STT research community, we release the corpus free for non-commercial use.