A Path Space Extension for Robust Light Transport Simulation
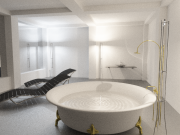
We propose a new sampling space for light transport simulation which allows to unify two popular algorithms with orthogonal strengths: unbiased Monte Carlo path integration and photon density estimation. Traditionally, unbiased Monte Carlo path integration has been considered the only approach for accurate light transport simulation. However, recent work in photon density estimation has demonstrated that there are several practical scene configurations where photon density estimation is more efficient and accurate. In order to take the best of both worlds without relying on a heuristic choice of the algorithms, we combine both algorithms through multiple importance sampling. Our contributions are two-fold: first, we introduce a path space extension that serves as a basis for a unified view of unbiased Monte Carlo path integration and photon density estimation. This extension gives us a mathematical ground for fully robust light transport simulation algorithms; second, we extend the theoretical analysis of provably good multiple importance sampling strategies by considering the presence of a density estimation method. This analysis leads to important conditions for obtaining a nearly optimal combination of unbiased Monte Carlo path integration and photon density estimation. We demonstrate that the resulting algorithm can robustly render many scene configurations that were previously considered intractable.