Motion-I2V: Consistent and Controllable Image-to-Video Generation with Explicit Motion Modeling
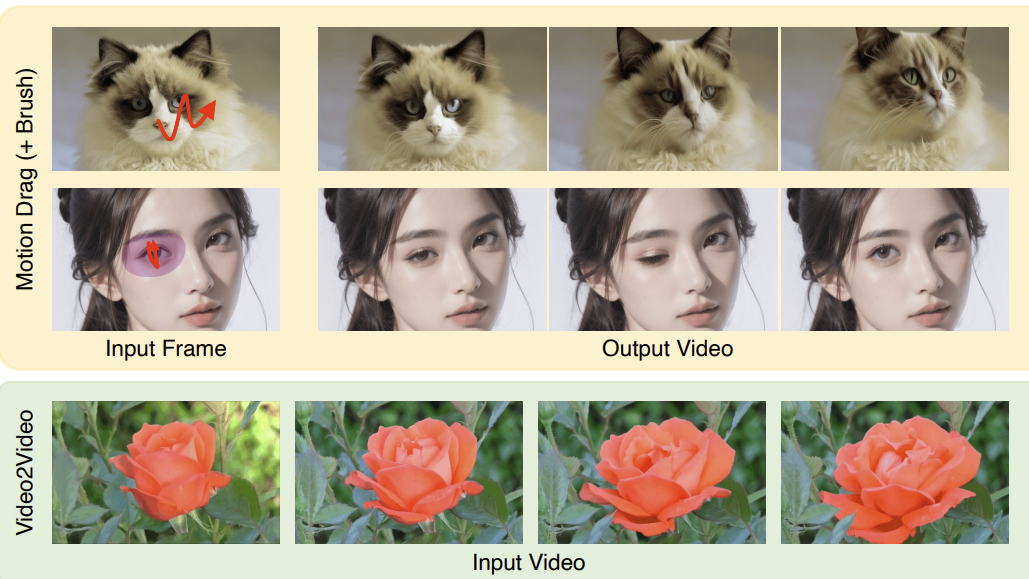
We introduce Motion-I2V, a novel framework for consistent and controllable image-to-video generation (I2V). In contrast to previous methods that directly learn the complicated image-to-video mapping, Motion-I2V factorizes I2V into two stages with explicit motion modeling. For the first stage, we propose a diffusion-based motion field predictor, which focuses on deducing the trajectories of the reference image's pixels. For the second stage, we propose motion-augmented temporal attention to enhance the limited 1-D temporal attention in video latent diffusion models. This module can effectively propagate reference image's feature to synthesized frames with the guidance of predicted trajectories from the first stage. Compared with existing methods, Motion-I2V can generate more consistent videos even at the presence of large motion and viewpoint variation. By training a sparse trajectory ControlNet for the first stage, Motion-I2V can support users to precisely control motion trajectories and motion regions with sparse trajectory and region annotations. This offers more controllability of the I2V process than solely relying on textual instructions. Additionally, Motion-I2V's second stage naturally supports zero-shot video-to-video translation. Both qualitative and quantitative comparisons demonstrate the advantages of Motion-I2V over prior approaches in consistent and controllable image-to-video generation. Please see our project page at this https URL.