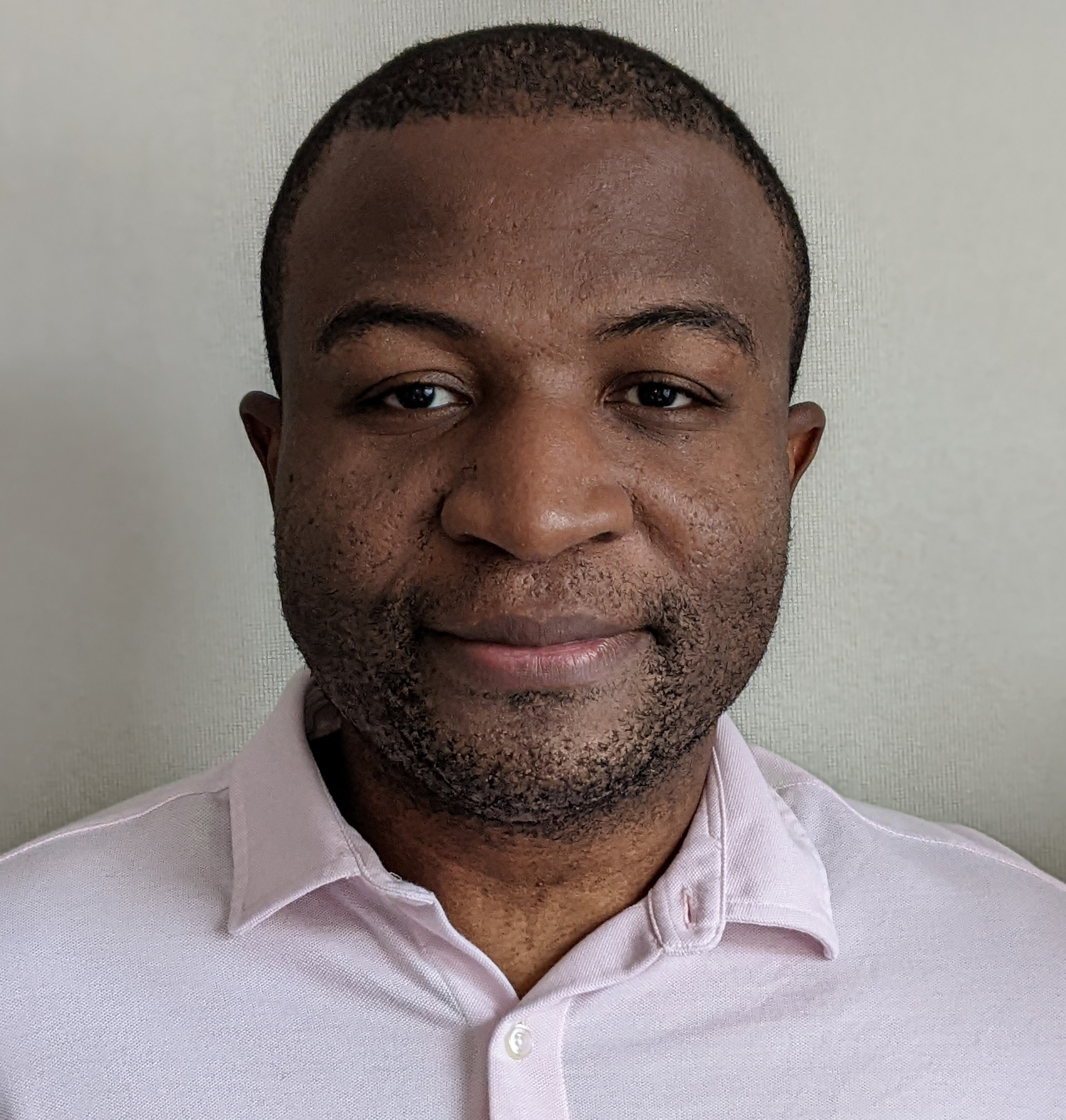
Thierry's work aims to optimize natural language processing (NLP) at the algorithm, hardware architecture, and solid-state layers. His research led to the creation of a novel and hardware-friendly encoding datatype, AdaptivFloat, which produces higher inference accuracies at low bit precision compared to many other prominent datatypes used in deep learning computations. Subsequently, Thierry led the development of a 16nm system-on-chip for on-device multi-domain NLP featuring hardware acceleration of attention-based DNNs with AdaptivFloat-based processing elements, and Bayesian based speech enhancement. Thierry also investigates to what extent the very dense, albeit stochastic, storage capabilities of emerging non-volatile memories can be exploited in satisfying the always-on and intermediate computing requirements of fully on-chip multi-task NLP.
Thierry Tambe is a PhD candidate in Electrical Engineering at Harvard University. His current research interests focus on designing algorithms, energy-efficient and high-performance hardware accelerators and systems for machine learning and natural language processing in particular. He also bears a keen interest in agile SoC design methodologies. Prior to debuting his doctoral studies, Thierry was a staff engineer at Intel in Hillsboro, Oregon, USA where he designed various analog/mixed-signal architectures for high-bandwidth memory and peripheral interfaces on Xeon and Xeon-Phi HPC SoCs. Thierry received a B.S. in 2010 and M.Eng. in 2012 in Electrical Engineering from Texas A&M University.