Riemannian Motion Policies
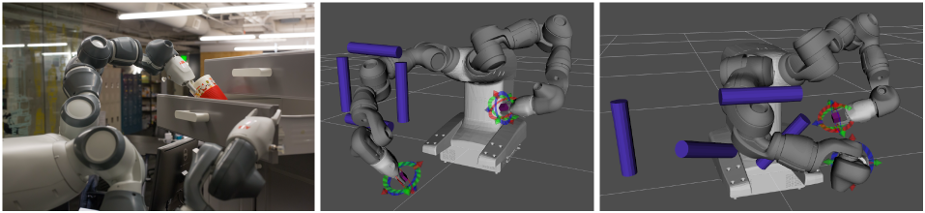
A new mathematical framework called Riemannian Motion Policies (RMPs) shapes a robot’s behavior. We derive optimal and practical tools for intuitively constructing policies, demonstrate the framework’s flexibility for distributed computation, use it to unify many previously distinct motion generation techniques, and demonstrate its performance on three dual arm manipulation platforms in both simulation and reality. RMPs achieve strong generalization both across many motion tasks and robots by being careful about the geometrical consistency of their operations in a way analogous to natural gradients from machine learning. We solve challenging collision avoidance problems entirely with local reactive policies, which results in instantaneous natural and adaptive motion with zero pre-planning and orders of magnitude effective speedups over traditional computationally complex planners.