Deep Learning-based Enhancement of Epigenomics Data with AtacWorks
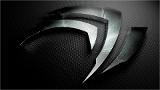
ATAC-seq is a widely-applied assay used to measure genome-wide chromatin accessibility; however, its ability to detect active regulatory regions can depend on the depth of sequencing coverage and the signal-to-noise ratio. Here we introduce AtacWorks, a deep learning toolkit to denoise sequencing coverage and identify regulatory peaks at base-pair resolution from low cell count, low-coverage, or low-quality ATAC-seq data. Models trained by AtacWorks can detect peaks from cell types not seen in the training data, and are generalizable across diverse sample preparations and experimental platforms. We demonstrate that AtacWorks enhances the sensitivity of single-cell experiments by producing results on par with those of conventional methods using ~10 times as many cells, and further show that this framework can be adapted to enable cross-modality inference of protein-DNA interactions. Finally, we establish that AtacWorks can enable new biological discoveries by identifying active regulatory regions associated with lineage priming in rare subpopulations of hematopoietic stem cells.