Motion reasoning for goal-based imitation learning
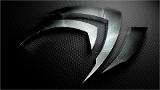
We address goal-based imitation learning, where the aim is to output the symbolic goal from a third-person video demonstration. This enables the robot to plan for execution and reproduce the same goal in a completely different environment. The key challenge is that the goal of a video demonstration is often ambiguous at the level of semantic actions. The human demonstrators might unintentionally achieve certain subgoals in the demonstrations with their actions. Our main contribution is to propose a motion reasoning framework that combines task and motion planning to disambiguate the true intention of the demonstrator in the video demonstration. This allows us to recognize the goals that cannot be disambiguated by previous action-based approaches. We evaluate our approach on a new dataset of 96 video demonstrations in a mockup kitchen environment. We show that our motion reasoning plays an important role in recognizing the actual goal of the demonstrator and improves the success rate by over 20%. We further show that by using the automatically inferred goal from the video demonstration, our robot is able to reproduce the same task in a real kitchen environment.
Publication Date
Published in
Research Area
External Links
Copyright
This material is posted here with permission of the IEEE. Internal or personal use of this material is permitted. However, permission to reprint/republish this material for advertising or promotional purposes or for creating new collective works for resale or redistribution must be obtained from the IEEE by writing to pubs-permissions@ieee.org.