Toward Sim-to-Real Directional Semantic Grasping
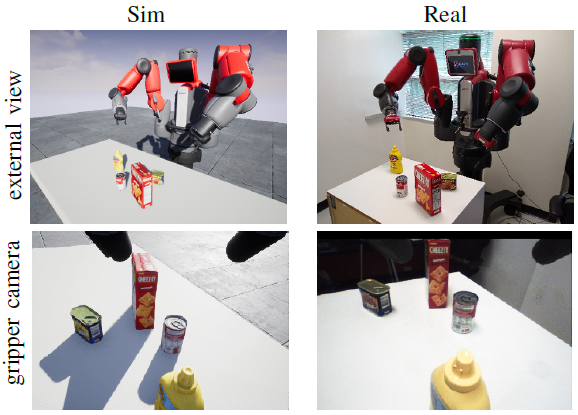
We address the problem of directional semantic grasping, that is, grasping a specific object from a specific direction. We approach the problem using deep reinforcement learning via a double deep Q-network (DDQN) that learns to map downsampled RGB input images from a wrist-mounted camera to Q-values, which are then translated into Cartesian robot control commands via the cross-entropy method (CEM). The network is learned entirely on simulated data generated by a custom robot simulator that models both physical reality (contacts) and perceptual quality (high-quality rendering). The reality gap is bridged using domain randomization. The system is an example of end-to-end (mapping input monocular RGB images to output Cartesian motor commands) grasping of objects from multiple pre-defined object-centric orientations, such as from the side or top. We show promising results in both simulation and the real world, along with some challenges faced and the need for future research in this area.