6-DOF Grasping for Target-driven Object Manipulation in Clutter
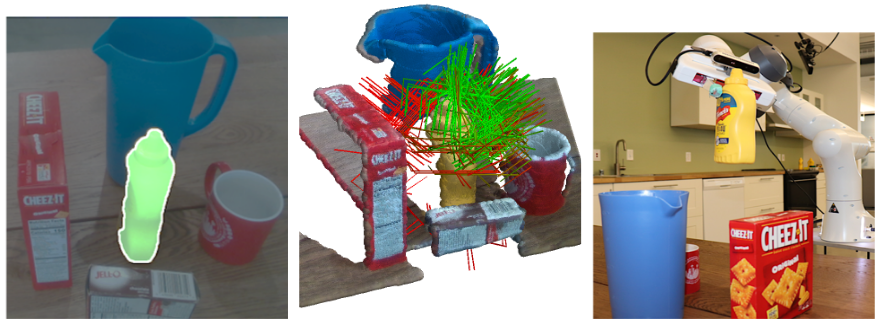
Grasping in cluttered environments is a fundamental but challenging robotic skill. It requires both reasoning about unseen object parts and potential collisions with the manipulator. Most existing data-driven approaches avoid this problem by limiting themselves to top-down planar grasps which is insufficient for many real-world scenarios and greatly limits possible grasps. We present a method that plans 6-DOF grasps for any desired object in a cluttered scene from partial point cloud observations. Our method achieves a grasp success of 80.3%, outperforming baseline approaches by 17.6% and clearing 9 cluttered table scenes (which contain 23 unknown objects and 51 picks in total) on a real robotic platform. By using our learned collision checking module, we can even reason about effective grasp sequences to retrieve objects that are not immediately accessible.
Finalist for ICRA 2020 Best Student & Best Manipulation Paper Award (http://www.icra2020.org/program/conference-awards)