Collaborative Interaction Models for Optimized Human-Robot Teamwork
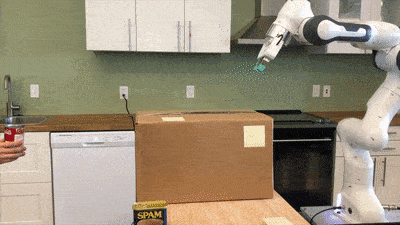
Effective human-robot collaboration requires informed anticipation. The robot must anticipate the human's actions, but also react quickly and intuitively when its predictions are wrong. The robot must plan its actions to account for the human's own plan, with the knowledge that the human's behavior will change based on what the robot actually does. This cyclical game of predicting a human's future actions and generating a corresponding motion plan is extremely difficult to model using standard techniques. In this work, we describe a novel Model Predictive Control (MPC)-based framework for finding optimal trajectories in a collaborative, multi-agent setting, in which we simultaneously plan for the robot while predicting the actions of its external collaborators. We use human-robot handovers to demonstrate that with a strong model of the collaborator, our framework produces fluid, reactive human-robot interactions in novel, cluttered environments. Our method efficiently generates coordinated trajectories, and achieves a high success rate in handover, even in the presence of significant sensor noise.
Publication Date
Research Area
Copyright
This material is posted here with permission of the IEEE. Internal or personal use of this material is permitted. However, permission to reprint/republish this material for advertising or promotional purposes or for creating new collective works for resale or redistribution must be obtained from the IEEE by writing to pubs-permissions@ieee.org.