RMPflow: A Geometric Framework for Generation of Multi-Task Motion Policies
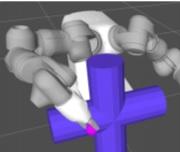
Generating robot motion for multiple tasks in dynamic environments is challenging, requiring an algorithm to respond reactively while accounting for complex nonlinear relationships between tasks. In this paper, we develop a novel policy synthesis algorithm, RMPflow, based on geometrically consistent transformations of Riemannian Motion Policies (RMPs). RMPs are a class of reactive motion policies that parameterize non-Euclidean behaviors as dynamical systems in intrinsically nonlinear task spaces. Given a set of RMPs designed for individual tasks, RMPflow can combine these policies to generate an expressive global policy, while simultaneously exploiting sparse structure for computational efficiency. We study the geometric properties of RMPflow and provide sufficient conditions for stability. Finally, we experimentally demonstrate that accounting for the natural Riemannian geometry of task policies can simplify classically difficult problems, such as planning through clutter on high-DOF manipulation systems.