NVIDIA NeMo Offline Speech Translation Systems for IWSLT 2022
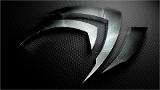
This paper provides an overview of NVIDIA NeMo’s speech translation systems for the IWSLT 2022 Offline Speech Translation Task. Our cascade system consists of 1) Conformer RNN-T automatic speech recognition model, 2) punctuation-capitalization model based on pre-trained T5 encoder, 3) ensemble of Transformer neural machine translation models fine-tuned on TED talks. Our end-to-end model has less parameters and consists of Conformer encoder and Transformer decoder. It relies on the cascade system by re-using its pre-trained ASR encoder and training on synthetic translations generated with the ensemble of NMT models. Our En->De cascade and end-to-end systems achieve 29.7 and 26.2 BLEU on the 2020 test set correspondingly, both outperforming the previous year’s best of 26 BLEU.