GPU-Accelerated Partially Linear Multiuser Detection for 5G and Beyond URLLC Systems
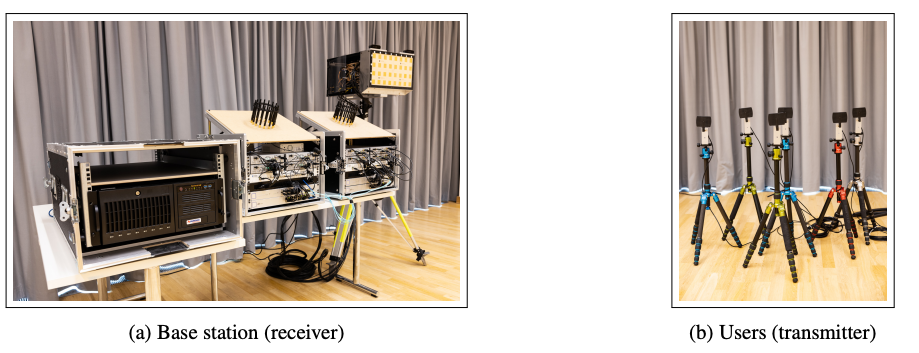
We have implemented a recently proposed partially linear multiuser detection algorithm in reproducing kernel Hilbert spaces (RKHSs) on a GPU-accelerated platform. Our proof of concept combines the robustness of linear detection and non-linear detection for the non-orthogonal multiple access (NOMA) based massive connectivity scenario. Mastering the computation of the vast number of inner products (which involve kernel evaluations) is a challenge in ultra-low latency (ULL) applications due to the sub-millisecond latency requirement. To address the issue, we propose a massively parallel implementation of the detection of user data in a received orthogonal frequency-division multiplexing (OFDM) radio frame. The result is a GPU-accelerated real-time OFDM receiver that enables detection latency of less than one millisecond that complies with the requirements of 5th generation (5G) and beyond ultra-reliable and low latency communications (URLLC) systems. Moreover, the parallelization and acceleration techniques explored and demonstrated in this study can be extended to many signal processing algorithms in Hilbert spaces, such as projection onto convex sets (POCS) and adaptive projected subgradient method (APSM) based algorithms. Results and comparisons with the state-of-the-art confirm the effectiveness of our approach.
Publication Date
Published in
External Links
Copyright
This material is posted here with permission of the IEEE. Internal or personal use of this material is permitted. However, permission to reprint/republish this material for advertising or promotional purposes or for creating new collective works for resale or redistribution must be obtained from the IEEE by writing to pubs-permissions@ieee.org.