Adaptive NN-based OFDM Receivers: Computational Complexity vs. Achievable Performance
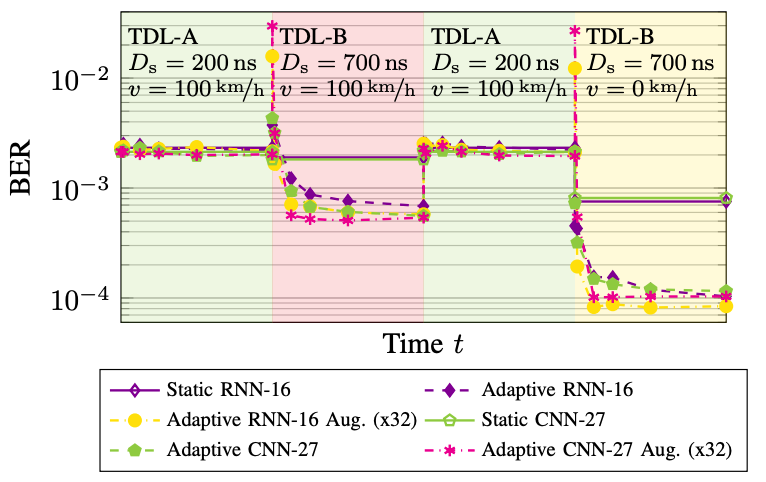
We revisit the design and retraining capabilities of neural network (NN)-based orthogonal frequency division multiplex (OFDM) receivers that combine channel estimation, equalization and soft-demapping for time-varying and frequency selective wireless channels. Attracted by the inherent advantages of small NNs in terms of computational complexity during inference and (re-)training, we first analyze the performance of different neural receiver architectures, including versions with reduced complexity. We observe, that such neural receivers with reduced complexity show an expected but graceful degradation in terms of their achievable bit error rate (BER) performance. Further, we focus on the adaptive retraining of site-specific NN-based receivers and show that performance losses due to a reduced number of parameters can be compensated by a continuous retraining of the receiver on-the-fly and that generalization can be achieved through adaptivity. Finally, we propose an improved retraining process via data augmentation and demonstrate a better performance and faster adaptation to current channel conditions.
Publication Date
Research Area
Copyright
This material is posted here with permission of the IEEE. Internal or personal use of this material is permitted. However, permission to reprint/republish this material for advertising or promotional purposes or for creating new collective works for resale or redistribution must be obtained from the IEEE by writing to pubs-permissions@ieee.org.