Towards Adaptive Digital Self-Interference Cancellation in Full-Duplex Wireless Transceivers: APSM vs. Neural Networks
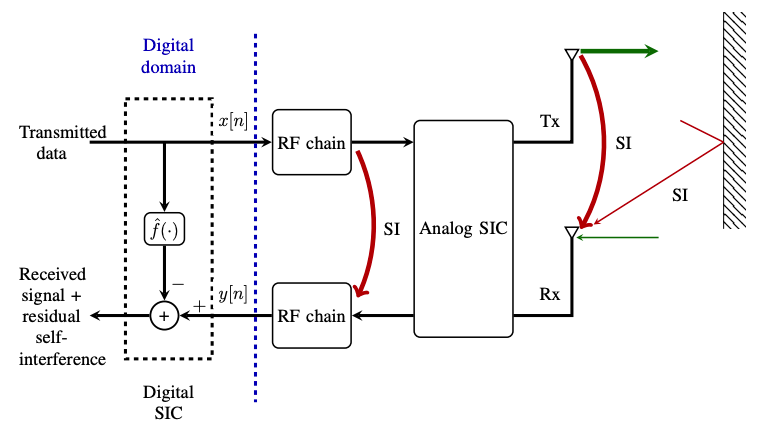
We investigate adaptive projected sub gradient method (APSM) and neural network (NN) machine learning techniques to address the challenge of digital self-interference cancellation in full-duplex communications. To this end, we compare both approaches in terms of their interference suppression capabilities, their computational complexity, and discuss their potential of continual training. Both approaches can take advantage of massively parallel processing in the digital domain, resulting in a significantly reduced end-to-end latency. Moreover, adaptive online training allows for tracking changes in dynamic wireless environments while compensating various practical impairments such as transceiver non-linearities. Our dedicated hardware setup enables us to study multiple scenarios and demonstrates the practicability of the algorithms in actual over-the-air deployments.
Publication Date
Copyright
This material is posted here with permission of the IEEE. Internal or personal use of this material is permitted. However, permission to reprint/republish this material for advertising or promotional purposes or for creating new collective works for resale or redistribution must be obtained from the IEEE by writing to pubs-permissions@ieee.org.