TTA-COPE: Test-Time Adaptation for Category-Level Object Pose Estimation
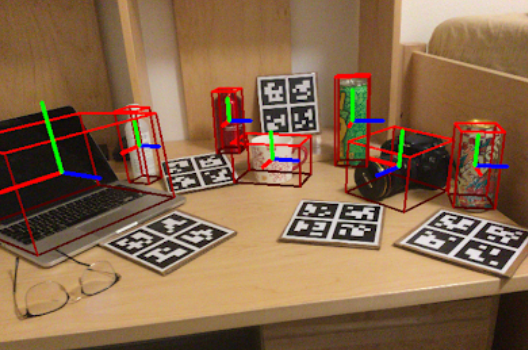
Test-time adaptation methods have been gaining attention recently as a practical solution for addressing source-to-target domain gaps by gradually updating the model without requiring labels on the target data. In this paper, we propose a method of test-time adaptation for category-level object pose estimation called TTA-COPE. We design a pose ensemble approach with a self-training loss using pose-aware confidence. Unlike previous unsupervised domain adaptation methods for category-level object pose estimation, our approach processes the test data in a sequential, online manner, and it does not require access to the source domain at runtime. Extensive experimental results demonstrate that the proposed pose ensemble and the self-training loss improve category-level object pose performance during test time under both semi-supervised and unsupervised settings.