ML-based Fault Injection for Autonomous Vehicles: A Case for Bayesian Fault Injection
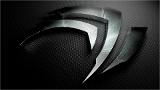
The safety and resilience of fully autonomous vehicles (AVs) are of significant concern, as exemplified by several headline-making accidents. While AV development today involves verification, validation, and testing, end-to-end assessment of AV systems under accidental faults in realistic driving scenarios has been largely unexplored. This paper presents DriveFI, a machine learning-based fault injection engine, which can mine situations and faults that maximally impact AV safety, as demonstrated on two industry-grade AV technology stacks (from NVIDIA and Baidu). For example, DriveFI found 561 safety-critical faults in less than 4 hours. In comparison, random injection experiments executed over several weeks could not find any safety-critical faults.
Publication Date
External Links
Uploaded Files
Copyright
This material is posted here with permission of the IEEE. Internal or personal use of this material is permitted. However, permission to reprint/republish this material for advertising or promotional purposes or for creating new collective works for resale or redistribution must be obtained from the IEEE by writing to pubs-permissions@ieee.org.