GPS: A Global Publish-Subscribe Model for Multi-GPU Memory Management
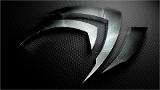
Suboptimal management of memory and bandwidth is one of the primary causes of low performance on systems comprising multiple GPUs. Existing memory management solutions like Unified Memory (UM) offer simplified programming but come at the cost of performance: applications can even exhibit slowdown with increasing GPU count due to their inability to leverage system resources effectively. To solve this challenge, we propose GPS, a HW/SW multi-GPU memory management technique that efficiently orchestrates inter-GPU communication using proactive data transfers. GPS offers the programmability advantage of multi-GPU shared memory with the performance of GPU-local memory. To enable this, GPS automatically tracks the data accesses performed by each GPU, maintains duplicate physical replicas of shared regions in each GPU’s local memory, and pushes updates to the replicas in all consumer GPUs. GPS is compatible within the existing NVIDIA GPU memory consistency model but takes full advantage of its relaxed nature to deliver high performance. We evaluate GPS in the context of a 4-GPU system with varying interconnects and show that GPS achieves an average speedup of 3.0× relative to the performance of a single GPU, outperforming the next best available multi-GPU memory management technique by 2.3× on average. In a 16-GPU system, using a future PCIe 6.0 interconnect, we demonstrate a 7.9× average strong scaling speedup over single-GPU performance, capturing 80% of the available opportunity.
Publication Date
Published in
Research Area
External Links
Uploaded Files
Awards
Copyright
Copyright by the Association for Computing Machinery, Inc. Permission to make digital or hard copies of part or all of this work for personal or classroom use is granted without fee provided that copies are not made or distributed for profit or commercial advantage and that copies bear this notice and the full citation on the first page. Copyrights for components of this work owned by others than ACM must be honored. Abstracting with credit is permitted. To copy otherwise, to republish, to post on servers, or to redistribute to lists, requires prior specific permission and/or a fee. Request permissions from Publications Dept, ACM Inc., fax +1 (212) 869-0481, or permissions@acm.org. The definitive version of this paper can be found at ACM's Digital Library http://www.acm.org/dl/.