Multilayer and Multimodal Fusion of Deep Neural Networks for Video Classification
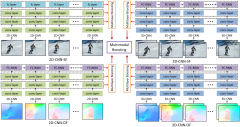
This paper presents a novel framework to combine multiple layers and modalities of deep neural networks for video classification. We first propose a multilayer strategy to simultaneously capture a variety of levels of abstraction and invariance in a network, where the convolutional and fully connected layers are effectively represented by the proposed feature aggregation methods. We further introduce a multimodal scheme that includes four highly complementary modalities to extract diverse static and dynamic cues at multiple temporal scales. In particular, for modeling the long-term temporal information, we propose a new structure, FC-RNN, to effectively transform pre-trained fully connected layers into recurrent layers. A robust boosting model is then introduced to optimize the fusion of multiple layers and modalities in a unified way. In the extensive experiments, we achieve state-of-the-art results on two public benchmark datasets: UCF101 and HMDB51.
Publication Date
Published in
Research Area
Uploaded Files
Copyright
Copyright by the Association for Computing Machinery, Inc. Permission to make digital or hard copies of part or all of this work for personal or classroom use is granted without fee provided that copies are not made or distributed for profit or commercial advantage and that copies bear this notice and the full citation on the first page. Copyrights for components of this work owned by others than ACM must be honored. Abstracting with credit is permitted. To copy otherwise, to republish, to post on servers, or to redistribute to lists, requires prior specific permission and/or a fee. Request permissions from Publications Dept, ACM Inc., fax +1 (212) 869-0481, or permissions@acm.org. The definitive version of this paper can be found at ACM's Digital Library http://www.acm.org/dl/.