Hand Pose Estimation via Latent 2.5 D Heatmap Regression
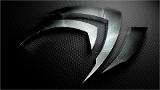
Estimating the 3D pose of a hand is an essential part of human-computer interaction. Estimating 3D pose using depth or multi- view sensors has become easier with recent advances in computer vision, however, regressing pose from a single RGB image is much less straight- forward. The main difficulty arises from the fact that 3D pose requires some form of depth estimates, which are ambiguous given only an RGB image. In this paper we propose a new method for 3D hand pose estima- tion from a monocular image through a novel 2.5D pose representation. Our new representation estimates pose up to a scaling factor, which can be estimated additionally if a prior of the hand size is given. We im- plicitly learn depth maps and heatmap distributions with a novel CNN architecture. Our system achieves state-of-the-art accuracy for 2D and 3D hand pose estimation on several challenging datasets in presence of severe occlusions.
Publication Date
Published in
Research Area
External Links
Copyright
This material is posted here with permission of the IEEE. Internal or personal use of this material is permitted. However, permission to reprint/republish this material for advertising or promotional purposes or for creating new collective works for resale or redistribution must be obtained from the IEEE by writing to pubs-permissions@ieee.org.