Separating Reflection and Transmission Images in the Wild
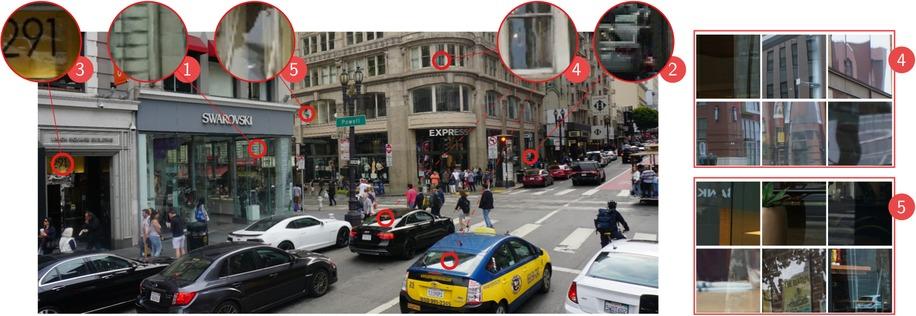
The reflections caused by common semi-reflectors, such as glass windows, can impact the performance of computer vision algorithms. State-of-the-art methods can remove reflections on synthetic data and in controlled scenarios. However, they are based on strong assumptions and do not generalize well to real-world images. Contrary to a common misconception, real-world images are challenging even when polarization information is used. We present ReflectNet, a deep learning approach to separate the reflected and the transmitted components of the recorded irradiance that explicitly uses the polarization properties of light. To train it, we introduce an accurate synthetic data generation pipeline, which simulates realistic reflections, including those generated by curved and non-ideal surfaces, non-static scenes, and high-dynamic-range scenes.
The code and the model for the paper are at: https://github.com/NVlabs/ReflectNet
The data can be downloaded here.