Routability-Driven Macro Placement with Embedded CNN-Based Prediction Model
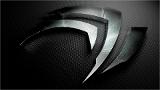
With the dramatic shrink of feature size and the advance of semiconductor technology nodes, numerous and complicated design rules need to be followed, and a chip design can only be taped-out after passing design rule check (DRC). The high design complexity seriously deteriorates design routability, which can be measured by the number of DRC violations after the detailed routing stage. In addition, a modern large-scaled design typically consists of many huge macros due to the wide use of intellectual properties (IPs). Empirically, the placement of these macros greatly determines routability, while there exists no effective cost metric to directly evaluate a macro placement because of the extremely high complexity and unpredictability of cell placement and routing. In this paper, we propose the first work of routability driven macro placement with deep learning. A convolutional neural network (CNN)-based routability prediction model is proposed and embedded into a macro placer such that a good macro placement w ith minimized DRC violations can be derived through a simulated annealing (SA) optimization process. Experimental results show the accuracy of the predictor and the effectiveness of the macro placer.
Publication Date
Published in
Copyright
This material is posted here with permission of the IEEE. Internal or personal use of this material is permitted. However, permission to reprint/republish this material for advertising or promotional purposes or for creating new collective works for resale or redistribution must be obtained from the IEEE by writing to pubs-permissions@ieee.org.