Buddy Compression: Enabling Larger Memory for Deep Learning and HPC Workloads on GPUs
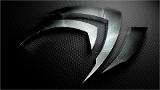
GPUs offer orders-of-magnitude higher memory bandwidth than traditional CPU-only systems. However, GPU device memory tends to be relatively small and the memory capacity can not be increased by the user. This paper describes Buddy Compression, a scheme to increase both the effective GPU memory capacity and bandwidth while avoiding the downsides of conventional memory-expanding strategies. Buddy Compression compresses GPU memory, splitting each compressed memory entry between high-speed device memory and a slower-but-larger disaggregated memory pool (or system memory). Highly-compressible memory entries can thus be accessed completely from device memory, while incompressible entries source their data using both on and off-device accesses. Increasing the effective GPU memory capacity enables us to run larger-memory-footprint HPC workloads and larger batch-sizes or models for DL workloads than current memory capacities would allow. We show that our solution achieves an average compression ratio of 2.2x on HPC workloads and 1.5x on DL workloads, with a slowdown of just 1~2%.