Content-Consistent Generation of Realistic Eyes with Style
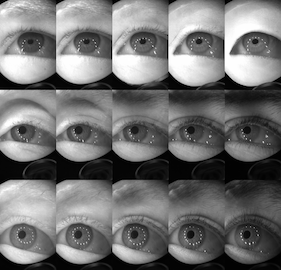
Accurately labeled real-world training data can be scarce, and hence recent works adapt, modify or generate images to boost target datasets. However, retaining relevant details from input data in the generated images is challenging and failure could be critical to the performance on the final task. In this work, we synthesize person-specific eye images that satisfy a given semantic segmentation mask (content), while following the style of a specified person from only a few reference images. We introduce two approaches, (a) one used to win the OpenEDS Synthetic Eye Generation Challenge at ICCV 2019, and (b) a principled approach to solving the problem involving simultaneous injection of style and content information at multiple scales. Our implementation is available at https://github.com/mcbuehler/Seg2Eye.
Publication Date
Published in
Uploaded Files
Award
Copyright
This material is posted here with permission of the IEEE. Internal or personal use of this material is permitted. However, permission to reprint/republish this material for advertising or promotional purposes or for creating new collective works for resale or redistribution must be obtained from the IEEE by writing to pubs-permissions@ieee.org.