Instant Quantization of neural networks using Monte Carlo Methods
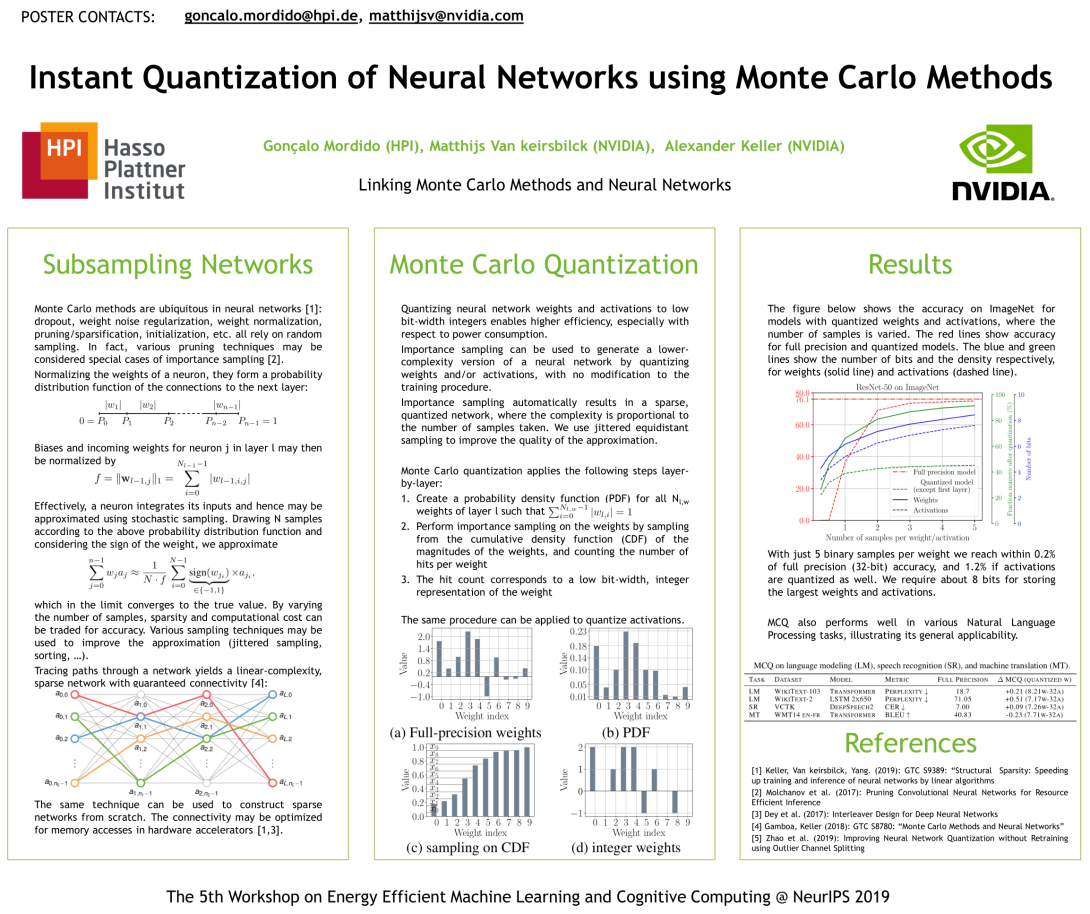
We propose Monte Carlo methods to leverage both sparsity and quantization to compress gradients of neural networks throughout training. On top of reducing the communication exchanged between multiple workers in a distributed setting, we also improve the computational efficiency of each worker. Our method, called Monte Carlo Gradient Quantization (MCGQ), shows faster convergence and higher performance than existing quantization methods on image classification and language modeling. Using both low-bit-width-quantization and high sparsity levels, our method more than doubles the rates of existing compression methods from 200xto 520xand 462xto more than 1200xon different language modeling tasks.