Novel View Synthesis of Dynamic Scenes with Globally Coherent Depths
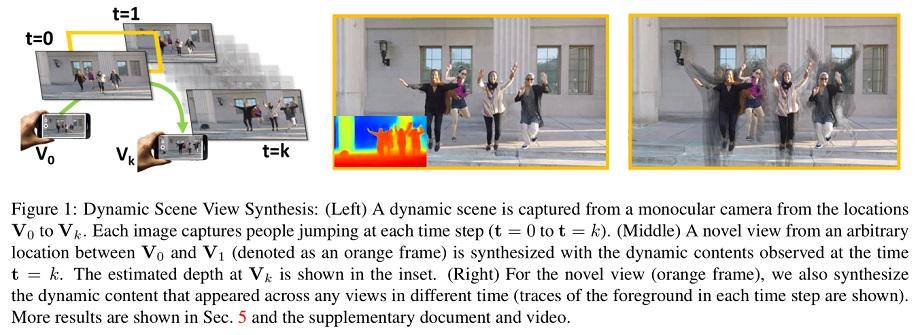
This paper presents a new method to synthesize an image from the arbitrary view and time given a collection of images of a dynamic scene. A key challenge for the synthesis arises from dynamic scene reconstruction where epipolar geometry does not apply to the local motion of dynamic contents. Our insight is that although its scale and quality is inconsistent with other views, the depth estimation from a single view can be used to reason about the geometry of the local motion. We propose to combine the depths from single view (DSV) and a view invariant depth reconstructed from multi-view stereo (DMV). We cast this problem as learning to correct the scale of DSV estimates, and to refine each depth with locally consistent motions between views to form a coherent depth estimation. We integrate these tasks into a depth fusion network in a self-supervised fashion. With the fused depth maps, we synthesize a photorealistic virtual view in a specific location and time using our deep blending network that completes the scene and renders the virtual view. We evaluate our method in depth estimation and view synthesis on a diverse real-world dynamic scenes and show the outstanding performance over existing methods.
Publication Date
Research Area
External Links
Copyright
This material is posted here with permission of the IEEE. Internal or personal use of this material is permitted. However, permission to reprint/republish this material for advertising or promotional purposes or for creating new collective works for resale or redistribution must be obtained from the IEEE by writing to pubs-permissions@ieee.org.