Learning Canonical Representations for Scene Graph to Image Generation
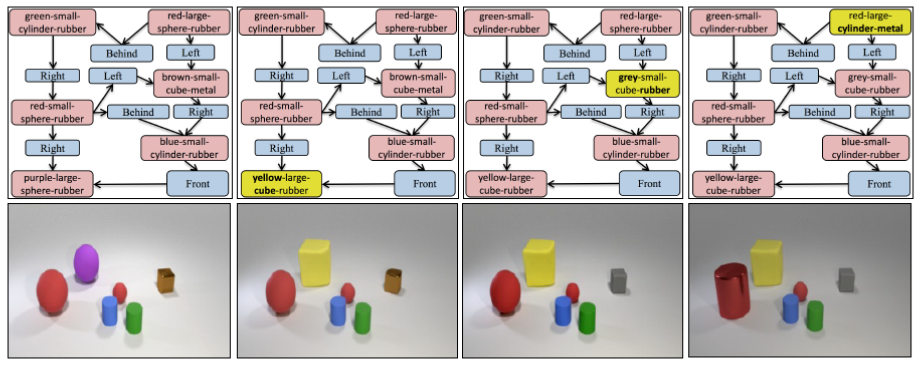
Generating realistic images of complex visual scenes becomes challenging when one wishes to control the structure of the generated images. Previous approaches showed that scenes with few entities can be controlled using scene graphs, but this approach struggles as the complexity of the graph (the number of objects and edges) increases. In this work, we show that one limitation of current methods is their inability to capture semantic equivalence in graphs. We present a novel model that addresses these issues by learning canonical graph representations from the data, resulting in improved image generation for complex visual scenes. Our model demonstrates improved empirical performance on large scene graphs, robustness to noise in the input scene graph, and generalization on semantically equivalent graphs. Finally, we show improved performance of the model on three different benchmarks: Visual Genome, COCO, and CLEVR.