From Generalized Zero-Shot Learning to Long-Tail with Class Descriptors
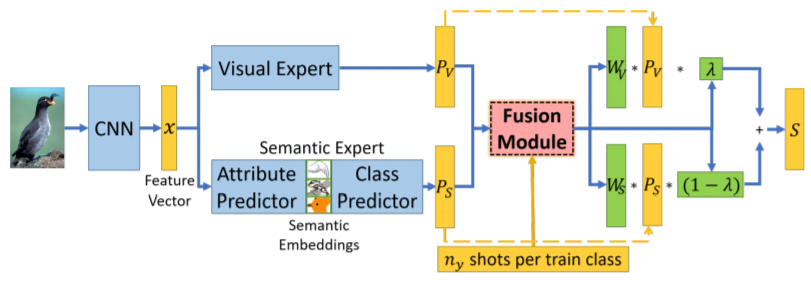
Real-world data is predominantly unbalanced and long-tailed, but deep models struggle to recognize rare classes in the presence of frequent classes. Often, classes can be accompanied by side information like textual descriptions, but it is not fully clear how to use them for learning with unbalanced long-tail data. Such descriptions have been mostly used in (Generalized) Zero-shot learning (ZSL), suggesting that ZSL with class descriptions may also be useful for long-tail distributions. We describe DRAGON, a late-fusion architecture for long-tail learning with class descriptors. It learns to (1) correct the bias towards head classes on a sample-by-sample basis; and (2) fuse information from class-descriptions to improve the tail-class accuracy. We also introduce new benchmarks CUB-LT, SUN-LT, AWA-LT for long-tail learning with class-descriptions, building on existing learning-with-attributes datasets and a version of Imagenet-LT with class descriptors. DRAGON outperforms state-of-the-art models on the new benchmark. It is also a new SoTA on existing benchmarks for GFSL with class descriptors (GFSL-d) and standard (vision-only) long-tailed learning ImageNet-LT, CIFAR-10, 100, and Places365.
Publication Date
Research Area
External Links
Copyright
This material is posted here with permission of the IEEE. Internal or personal use of this material is permitted. However, permission to reprint/republish this material for advertising or promotional purposes or for creating new collective works for resale or redistribution must be obtained from the IEEE by writing to pubs-permissions@ieee.org.