Fair and Comprehensive Benchmarking of Machine Learning Processing Chips
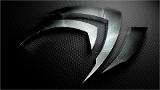
With the rise of custom silicon chips for AI acceleration, fair and comprehensive benchmarking of hardware innovations has become increasingly important. While benchmarking at the application- and system-level provides the most complete picture of tradeoffs across multiple design dimensions, this can hide the impact of innovations at lower levels. Moreover, system-level benchmarking is not always feasible, especially for academic and industrial research chips. Benchmarking machine learning chips at lower abstraction levels is therefore still useful and common practice, making use of architecture- or circuit-level metrics. Yet the selection of good metrics and benchmarking conditions is critical, as these strongly influence the correlation between the observed performance benefits and the final system-level benefits. This article provides an overview of benchmarking strategies at different abstraction levels, and discusses best practices and pitfalls to be avoided. We then propose to combine this diligent benchmarking at the appropriate individual abstraction level, with careful extrapolation to the system-level to also gauge impact at that topmost level. While the article focuses on neural network inference workloads, many guidelines discussed here are applicable to the broader class of machine learning chips.
Publication Date
Published in
Research Area
Copyright
This material is posted here with permission of the IEEE. Internal or personal use of this material is permitted. However, permission to reprint/republish this material for advertising or promotional purposes or for creating new collective works for resale or redistribution must be obtained from the IEEE by writing to pubs-permissions@ieee.org.