Citrinet: Closing the Gap between Non-Autoregressive and Autoregressive End-to-End Models for Automatic Speech Recognition
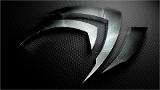
We propose Citrinet - a new end-to-end convolutional Connectionist Temporal Classification (CTC) based automatic speech recognition (ASR) model. Citrinet is deep residual neural model which uses 1D time-channel separable convolutions combined with sub-word encoding and squeeze-and-excitation. The resulting architecture significantly reduces the gap between non-autoregressive and sequence-to-sequence and transducer models. We evaluate Citrinet on LibriSpeech, TED-LIUM2, AISHELL-1 and Multilingual LibriSpeech (MLS) English speech datasets. Citrinet accuracy on these datasets is close to the best autoregressive Transducer models.