Controlling graph dynamics with reinforcement learning and graph neural networks
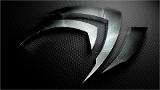
We consider the problem of controlling a partially-observed dynamic process on a graph by a limited number of interventions. This problem naturally arises in contexts such as scheduling virus tests to curb an epidemic; targeted marketing in order to promote a product; and manually inspecting posts to detect fake news spreading on social networks. We formulate this setup as a sequential decision problem over a temporal graph process. In face of an exponential state space, combinatorial action space and partial observability, we design a novel tractable scheme to control dynamical processes on temporal graphs. We successfully apply our approach to two popular problems that fall into our framework: prioritizing which nodes should be tested in order to curb the spread of an epidemic, and influence maximization on a graph.