Self-Supervised Object Detection via Generative Image Synthesis
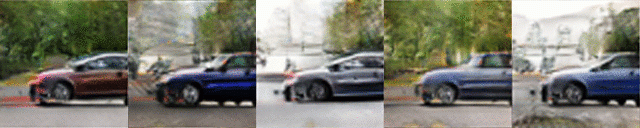
We present SSOD – the first end-to-end analysis-by-synthesis framework with controllable GANs for the task of self-supervised object detection. We use collections of real-world images without bounding box annotations to learn to synthesize and detect objects. We leverage controllable GANs to synthesize images with pre-defined object properties and use them to train object detectors. We propose a tight end-to-end coupling of the synthesis and detection networks to optimally train our system. Finally, we also propose a method to optimally adapt SSOD to an intended target data without requiring labels for it. For the task of car detection, on the challenging KITTI and Cityscapes datasets, we show that SSOD outperforms the prior state-of-the-art purely image-based self-supervised object detection method Wetectron. Even without requiring any 3DCAD assets, it also surpasses the state-of-the-art rendering-based method Meta-Sim2. Our work advances the field of self-supervised object detection by introducing a successful new paradigm of using controllable GAN-based image synthesis for it and by significantly improving the base-line accuracy of the task.