Detecting Viewer-Perceived Intended Vector Sketch Connectivity
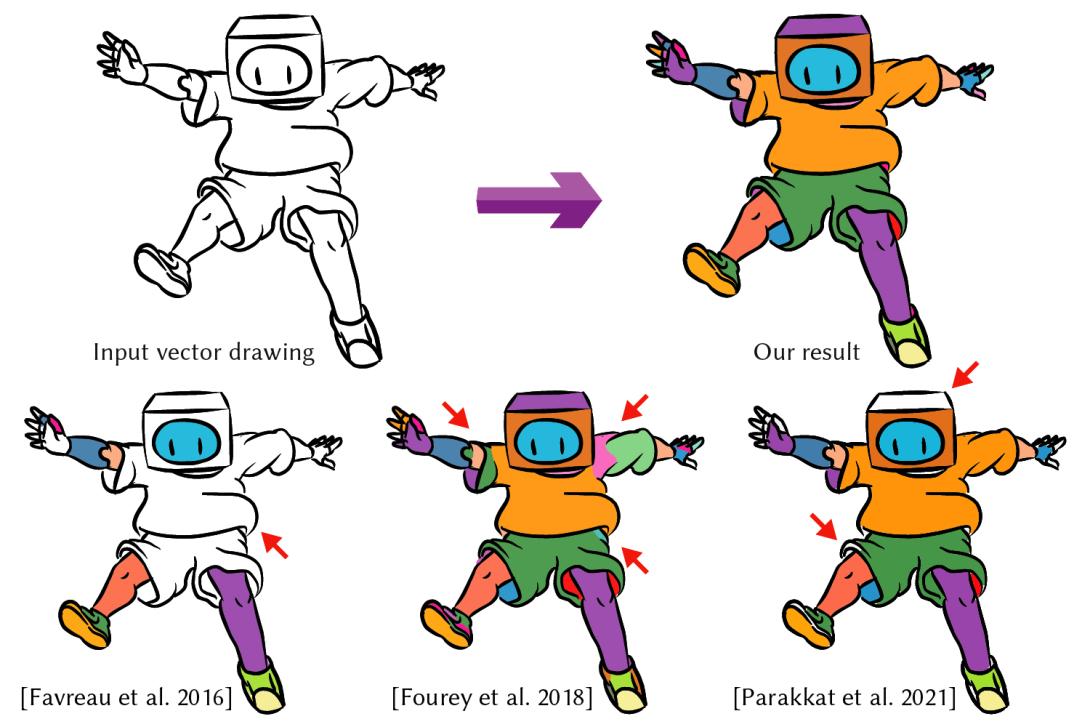
Many sketch processing applications target precise vector drawings with accurately specified stroke intersections, yet free-form artist drawn sketches are typically inexact: strokes that are intended to intersect often stop short of doing so. While human observers easily perceive the artist intended stroke connectivity, manually, or even semi-manually, correcting drawings to generate correctly connected outputs is tedious and highly time consuming.
We propose a novel, robust algorithm that extracts viewer-perceived stroke connectivity from inexact free-form vector drawings by leveraging observations about local and global factors that impact human perception of inter-stroke connectivity. We employ the identified local cues to train classifiers that assess the likelihood that pairs of strokes are perceived as forming end-to-end or T- junctions based on local context. We then use these classifiers within an incremental framework that combines classifier provided likelihoods with a more global, contextual and closure-based, analysis. We demonstrate our method on over 95 diversely sourced inputs, and validate it via a series of perceptual studies; participants prefer our outputs over the closest alternative by a factor of 9 to 1.
Publication Date
Published in
External Links
Uploaded Files
Copyright
Permission to make digital or hard copies of all or part of this work for personal or classroom use is granted without fee provided that copies are not made or distributed for profit or commercial advantage and that copies bear this notice and the full citation on the first page. Copyrights for components of this work owned by others than the author(s) must be honored. Abstracting with credit is permitted. To copy otherwise, or republish, to post on servers or to redistribute to lists, requires prior specific permission and/or a fee. Request permissions from permissions@acm.org. © 2022 Copyright held by the owner/author(s). Publication rights licensed to ACM. 0730-0301/2022/7-ART1 $15.00 https://doi.org/10.1145/3528223.3530097