A Compact End-to-End Model with Local and Global Context for Spoken Language Identification
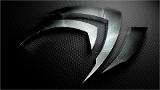
We introduce TitaNet-LID, a compact end-to-end neural network for Spoken Language Identification (LID) that is based on the ContextNet architecture. TitaNet-LID employs 1D depth-wise separable convolutions and Squeeze-and-Excitation layers to effectively capture local and global context within an utterance. Despite its small size, TitaNet-LID achieves performance similar to state-of-the-art models on the VoxLingua107 dataset while being 10 times smaller. Furthermore, it can be easily adapted to new acoustic conditions and unseen languages through simple fine-tuning, achieving a state-of-the-art accuracy of 88.2% on the FLEURS benchmark. Our model is scalable and can achieve a better trade-off between accuracy and speed. TitaNet-LID performs well even on short utterances less than 5s in length, indicating its robustness to input length.