DREAM-GAN: Advancing DREAMPlace towards Commercial-Quality using Generative Adversarial Learning
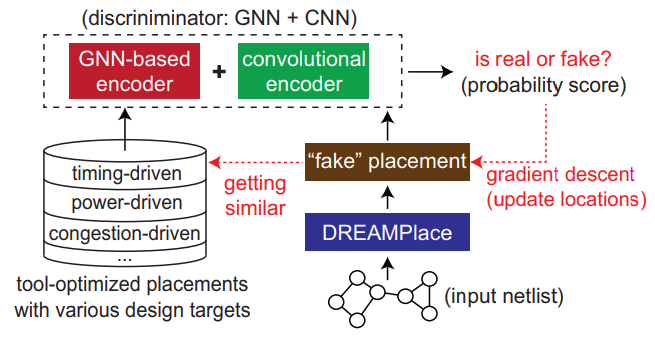
DREAMPlace is a renowned open-source placer that provides GPUacceleratable infrastructure for placements of Very-Large-ScaleIntegration (VLSI) circuits. However, due to its limited focus on wirelength and density, existing placement solutions of DREAMPlace are not applicable to industrial design flows. To improve DREAMPlace towards commercial-quality without knowing the black-boxed algorithms of the tools, in this paper, we present DREAM-GAN, a placement optimization framework that advances DREAMPlace using generative adversarial learning. At each placement iteration, aside from optimizing the wirelength and density objectives of the vanilla DREAMPlace, DREAM-GAN computes and optimizes a differentiable loss that denotes the similarity score between the underlying placement and the tool-generated placements in commercial databases. Experimental results on 5 commercial and OpenCore designs using an industrial design flow implemented by Synopsys ICC2 not only demonstrate that DREAM-GAN significantly improves the vanilla DREAMPlace at the placement stage across each benchmark, but also show that the improvements last firmly to the post-route stage, where we observe improvements by up to 8.3% in wirelength and 7.4% in total power
Publication Date
Published in
Uploaded Files
Copyright
Copyright by the Association for Computing Machinery, Inc. Permission to make digital or hard copies of part or all of this work for personal or classroom use is granted without fee provided that copies are not made or distributed for profit or commercial advantage and that copies bear this notice and the full citation on the first page. Copyrights for components of this work owned by others than ACM must be honored. Abstracting with credit is permitted. To copy otherwise, to republish, to post on servers, or to redistribute to lists, requires prior specific permission and/or a fee. Request permissions from Publications Dept, ACM Inc., fax +1 (212) 869-0481, or permissions@acm.org. The definitive version of this paper can be found at ACM's Digital Library http://www.acm.org/dl/.