Synthesizing Physical Character-Scene Interactions
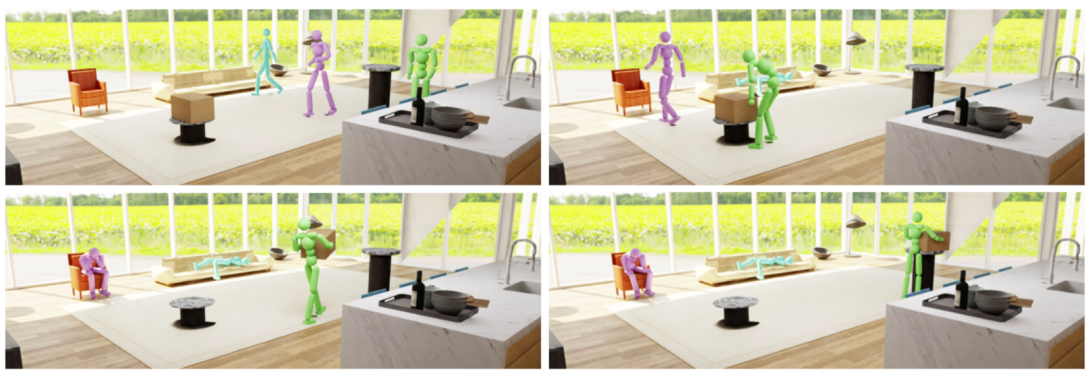
In this work, we present a system that uses adversarial imitation learning and reinforcement learning to train physically-simulated characters that perform scene interaction tasks in a natural and life-like manner. Our method learns scene interaction behaviors from large unstructured motion datasets, without manual annotation of the motion data. These scene interactions are learned using an adversarial discriminator that evaluates the realism of a motion within the context of a scene. We demonstrate the effectiveness of our approach through three challenging scene interaction tasks: carrying, sitting, and lying down, which require coordination of a character's movements in relation to objects in the environment. Our policies learn to seamlessly transition between different behaviors like idling, walking, and sitting.