Fugatto 1 - Foundational Generative Audio Transformer Opus 1
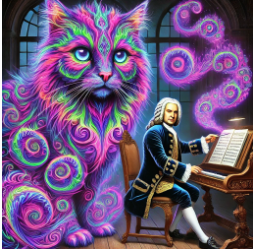
Fugatto is a versatile audio synthesis and transformation model capable of following
free-form text instructions with optional audio inputs. While large language
models (LLMs) trained with text on a simple next-token prediction objective can
learn to infer instructions directly from the data, models trained solely on audio
data lack this capacity. This is because audio data does not inherently contain the
instructions that were used to generate it. To overcome this challenge, we introduce
a specialized dataset generation approach optimized for producing a wide range of
audio generation and transformation tasks, ensuring the data reveals meaningful
relationships between audio and language. Another challenge lies in achieving
compositional abilities – such as combining, interpolating between, or negating
instructions – using data alone. To address it, we propose ComposableART, an
inference-time technique that extends classifier-free guidance to compositional
guidance. It enables the seamless and flexible composition of instructions, leading
to highly customizable audio outputs outside the training distribution. Our evaluations
across a diverse set of tasks demonstrate that Fugatto performs competitively
with specialized models, while ComposableART enhances its sonic palette and
control over synthesis. Most notably, we highlight our framework’s ability to
synthesize emergent sounds – sonic phenomena that transcend conventional audio
generation – unlocking new creative possibilities. Demo Website.