Improving Deep Stereo Network Generalization with Geometric Priors
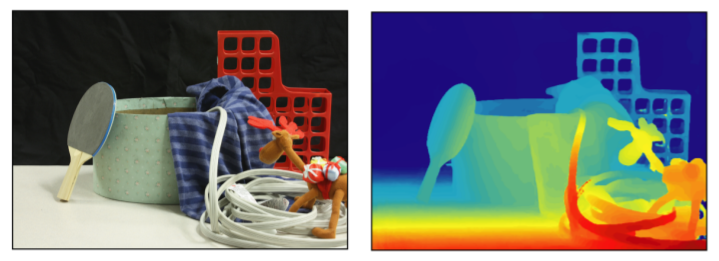
End-to-end deep learning methods have advanced stereo vision in recent years and obtained excellent results when the training and test data are similar. However, large datasets of diverse real-world scenes with dense ground truth are difficult to obtain and currently not publicly available to the research community. As a result, many algorithms rely on small real-world datasets of similar scenes or synthetic datasets, but end-to-end algorithms trained on such datasets often generalize poorly to different images that arise in real-world applications. As a step towards addressing this problem, we propose to incorporate prior knowledge of scene geometry into an end-to-end stereo network to help networks generalize better. For a given network, we explicitly add a gradient-domain smoothness prior and occlusion reasoning into the network training, while the architecture remains unchanged during inference. Experimentally, we show consistent improvements if we train on synthetic datasets and test on the Middlebury (real images) dataset. Noticeably, we improve PSM-Net accuracy on Middlebury from 5.37 MAE to 3.21 MAE without sacrificing speed.