Efficient Multi-GPU Shared Memory via Automatic Optimization of Fine-Grained Transfers
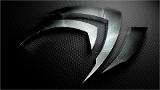
Despite continuing research into inter-GPU communication mechanisms, extracting performance from multi-GPU systems remains a significant challenge. Inter-GPU communication via bulk DMA-based transfers exposes data transfer latency on the GPU’s critical execution path because these large transfers are logically interleaved between compute kernels. Conversely, fine-grained peer-to-peer memory accesses during kernel execution lead to memory stalls that can exceed the GPUs’ ability to cover these operations via multi-threading. Worse yet, these sub-cacheline transfers are highly inefficient on current inter-GPU interconnects. To remedy these issues, we propose PROACT, a system enabling remote memory transfers with the programmability and pipeline advantages of peer-to-peer stores, while achieving interconnect efficiency that rivals bulk DMA transfers. Combining compile-time instrumentation with fine-grain tracking of data block readiness within each GPU, PROACT enables interconnect-friendly data transfers while hiding the transfer latency via pipelining during kernel execution. This work describes both hardware and software implementations of PROACT and demonstrates the effectiveness of a PROACT software prototype on three generations of GPU hardware and interconnects. Achieving near-ideal interconnect efficiency, PROACT realizes a mean speedup of 3.0x over single-GPU performance for 4-GPU systems, capturing 83% of available performance opportunity. On a 16-GPU NVIDIA DGX-2 system, we demonstrate an 11.0x average strong-scaling speedup over single-GPU performance, 5.3x better than a bulk DMA-based approach.
Publication Date
External Links
Uploaded Files
Copyright
This material is posted here with permission of the IEEE. Internal or personal use of this material is permitted. However, permission to reprint/republish this material for advertising or promotional purposes or for creating new collective works for resale or redistribution must be obtained from the IEEE by writing to pubs-permissions@ieee.org.