AutoDMP: Automated DREAMPlace-based Macro Placement
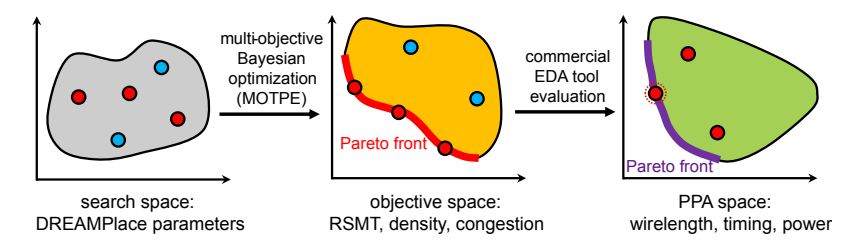
Macro placement is a critical very large-scale integration (VLSI) physical design problem that significantly impacts the design powerperformance-area (PPA) metrics. This paper proposes AutoDMP, a methodology that leverages DREAMPlace, a GPU-accelerated placer, to place macros and standard cells concurrently in conjunction with automated parameter tuning using a multi-objective hyperparameter optimization technique. As a result, we can generate high-quality predictable solutions, improving the macro placement quality of academic benchmarks compared to baseline results generated from academic and commercial tools. AutoDMP is also computationally efficient, optimizing a design with 2.7 million cells and 320 macros in 3 hours on a single NVIDIA DGX Station A100. This work demonstrates the promise and potential of combining GPU-accelerated algorithms and ML techniques for VLSI design automation
Publication Date
Published in
External Links
Uploaded Files
Copyright
Copyright by the Association for Computing Machinery, Inc. Permission to make digital or hard copies of part or all of this work for personal or classroom use is granted without fee provided that copies are not made or distributed for profit or commercial advantage and that copies bear this notice and the full citation on the first page. Copyrights for components of this work owned by others than ACM must be honored. Abstracting with credit is permitted. To copy otherwise, to republish, to post on servers, or to redistribute to lists, requires prior specific permission and/or a fee. Request permissions from Publications Dept, ACM Inc., fax +1 (212) 869-0481, or permissions@acm.org. The definitive version of this paper can be found at ACM's Digital Library http://www.acm.org/dl/.