Reinforcement Learning Guided Detailed Routing for Custom Circuits
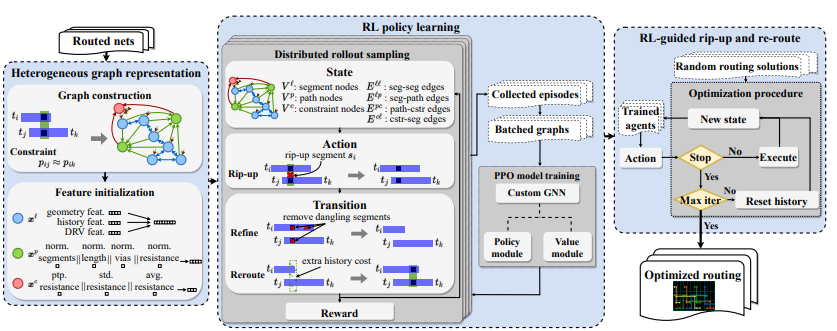
Detailed routing is the most tedious and complex procedure in design automation and has become a determining factor in layout automation in advanced manufacturing nodes. Despite continuing advances in custom integrated circuit (IC) routing research, industrial custom layout flows remain heavily manual due to the high complexity of the custom IC design problem. Besides conventional design objectives such as wirelength minimization, custom detailed routing must also accommodate additional constraints (e.g., path-matching) across the analog/mixed-signal (AMS) and digital domains, making an already challenging procedure even more so. This paper presents a novel detailed routing framework for custom circuits that leverages deep reinforcement learning to optimize routing patterns while considering custom routing constraints and industrial design rules. Comprehensive post-layout analyses based on industrial designs demonstrate the effectiveness of our framework in dealing with the specified constraints and producing sign-off-quality routing solutions.
Publication Date
Published in
External Links
Uploaded Files
Copyright
Copyright by the Association for Computing Machinery, Inc. Permission to make digital or hard copies of part or all of this work for personal or classroom use is granted without fee provided that copies are not made or distributed for profit or commercial advantage and that copies bear this notice and the full citation on the first page. Copyrights for components of this work owned by others than ACM must be honored. Abstracting with credit is permitted. To copy otherwise, to republish, to post on servers, or to redistribute to lists, requires prior specific permission and/or a fee. Request permissions from Publications Dept, ACM Inc., fax +1 (212) 869-0481, or permissions@acm.org. The definitive version of this paper can be found at ACM's Digital Library http://www.acm.org/dl/.