ATT3D: Amortized Text-To-3D Object Synthesis
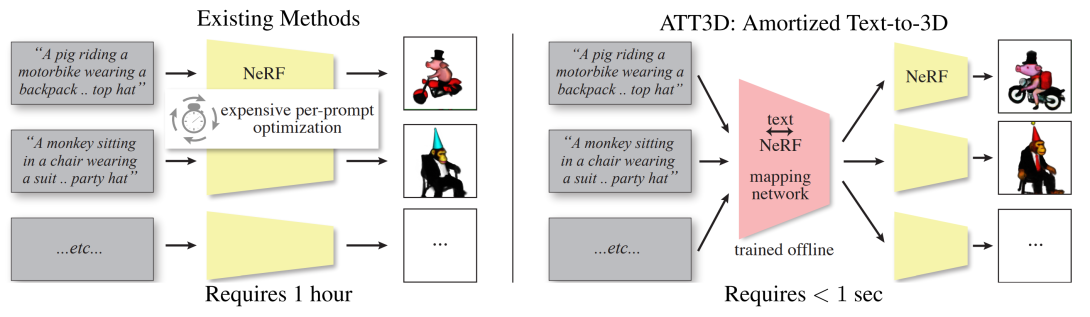
Text-to-3D modeling has seen exciting progress by combining generative text-to-image models with image-to-3D methods like Neural Radiance Fields. DreamFusion recently achieved high-quality results but requires a lengthy, per-prompt optimization to create 3D objects. To address this, we amortize optimization over text prompts by training on many prompts simultaneously with a unified model, instead of separately. With this, we share computation across a prompt set, training in less time than per-prompt optimization. Our framework - Amortized Text-to-3D (ATT3D) - enables sharing of knowledge between prompts to generalize to unseen setups and smooth interpolations between text for novel assets and simple animations.