Cosmos Transfer 1: World-to-World Transfer with Adaptive Multi-Control for Physical AI
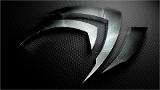
Physical AI systems rely on multi-modal sensory inputs to perceive and interact with the real world, yet these inputs often suffer from modality-specific biases, incomplete coverage, and perceptual gaps that hinder robust decision-making and generalization. To address this, we introduce Cosmos Transer, a World-to-World Transer model designed to bridge the perceptual divide between simulated and real-world environments, with a particular focus on Sim2REal transfer. Cosmos Transer leverages adaptive multi-ControlNets, each trained to condition on distinct world representations, such as semantic maps, depth, and edge information. At inference time, these ControlNets are fused via spatiotemporal control maps, which can either be specified for task-specific needs or dynamically optimized through learning, enabling flexible and context-aware synthesis of realistic world representations. We evaluate Cosmos Transfer in both a general-purpose World-to-World transfer setting and the autonomous driving domain, demonstrating its ability to integrate diverse simulated worlds into coherent, controllable, and adaptable representations of the physical world. Extensive quantitative and qualitative experiments show that Cosmos Transfer significantly enhances generalization, adaptability, and realism in downstream tasks. To accelerate future research in Physical AI, we release our models, inference pipeline, and fine-tuning code under the NVIDIA Open Model License at https://github.com/NVIDIA/Cosmos.