ꟻLIP: A Difference Evaluator for Alternating Images
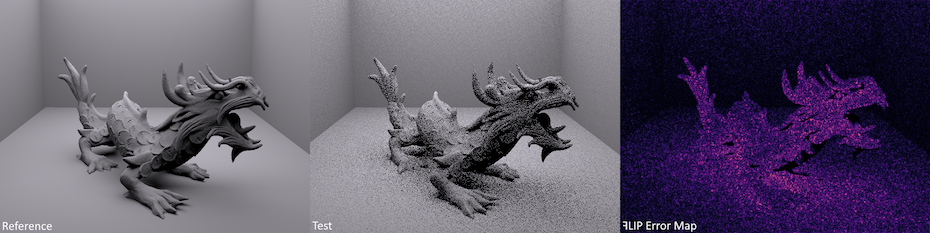
Abstract: Image quality measures are becoming increasingly important in the field of computer graphics. For example, there is currently a major focus on generating photorealistic images in real time by combining path tracing with denoising, for which such quality assessment is integral. We present ꟻLIP, which is a difference evaluator with a particular focus on the differences between rendered images and corresponding ground truths. Our algorithm produces a map that approximates the difference perceived by humans when alternating between two images. ꟻLIP is a combination of modified existing building blocks, and the net result is surprisingly powerful. We have compared our work against a wide range of existing image difference algorithms and we have visually inspected over a thousand image pairs that were either retrieved from image databases or generated in-house. We also present results of a user study which indicate that our method performs substantially better, on average, than the other algorithms.To facilitate the use of ꟻLIP, we provide source code in C++, CUDA, MATLAB, NumPy/SciPy, and PyTorch.
Please check out our gallery of images and error maps.
Hindsight: in the paper we advocated for the weighted median, computed from the weighted histogram, but this is not ideal. If a single number is to be used, then we recommend using the mean ꟻLIP value instead.
Errata:
p. 10: "... followed by division by 100" should be "... followed by division by 116."
High Dynamic Range (HDR) images: This version of ꟻLIP handles only low dynamic range images. If you wish to evaluate errors in HDR images, confer the paper
Visualizing Errors in Rendered High Dynamic Range Images, where a version of ꟻLIP that handles HDR images is introduced.
How to write an ꟻ:
HTML: ꟻ
Unicode: fileformat.info
LaTeX: \usepackage{mathtools} \usepackage{xspace} \newcommand{\FLIP}{\protect\reflectbox{F}LIP\xspace}
Publication Date
Published in
Research Area
Uploaded Files
Award
Copyright
Copyright by the Association for Computing Machinery, Inc. Permission to make digital or hard copies of part or all of this work for personal or classroom use is granted without fee provided that copies are not made or distributed for profit or commercial advantage and that copies bear this notice and the full citation on the first page. Copyrights for components of this work owned by others than ACM must be honored. Abstracting with credit is permitted. To copy otherwise, to republish, to post on servers, or to redistribute to lists, requires prior specific permission and/or a fee. Request permissions from Publications Dept, ACM Inc., fax +1 (212) 869-0481, or permissions@acm.org. The definitive version of this paper can be found at ACM's Digital Library http://www.acm.org/dl/.