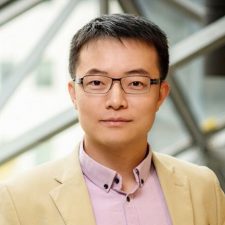
Song Han's research interest is efficient deep learning computing. He received his PhD degree from Stanford University advised by Prof. Bill Dally. He is also an Associate Professor at MIT (songhan.mit.edu). Song proposed the “Deep Compression” technique that is widely used for efficient AI, and “Efficient Inference Engine” that first brought weight sparsity to modern AI accelerator design. His team’s work on hardware-aware neural architecture search (once-for-all network) enables users to design, optimize, shrink and deploy AI models to resource-constrained hardware devices, receiving the first place in many low-power computer vision contests in flagship AI conferences. He pioneered the TinyML research that brings deep learning to IoT devices, enabling learning on the edge. Song received best paper awards at ICLR and FPGA. Song was named “35 Innovators Under 35” by MIT Technology Review for his contribution on “deep compression” technique that “lets powerful artificial intelligence (AI) programs run more efficiently on low-power mobile devices.” Song received the NSF CAREER Award for “efficient algorithms and hardware for accelerated machine learning”, the IEEE “AIs 10 to Watch: The Future of AI” award, and the Sloan Research Fellowship.