A 17–95.6 TOPS/W Deep Learning Inference Accelerator with Per-Vector Scaled 4-bit Quantization for Transformers in 5nm
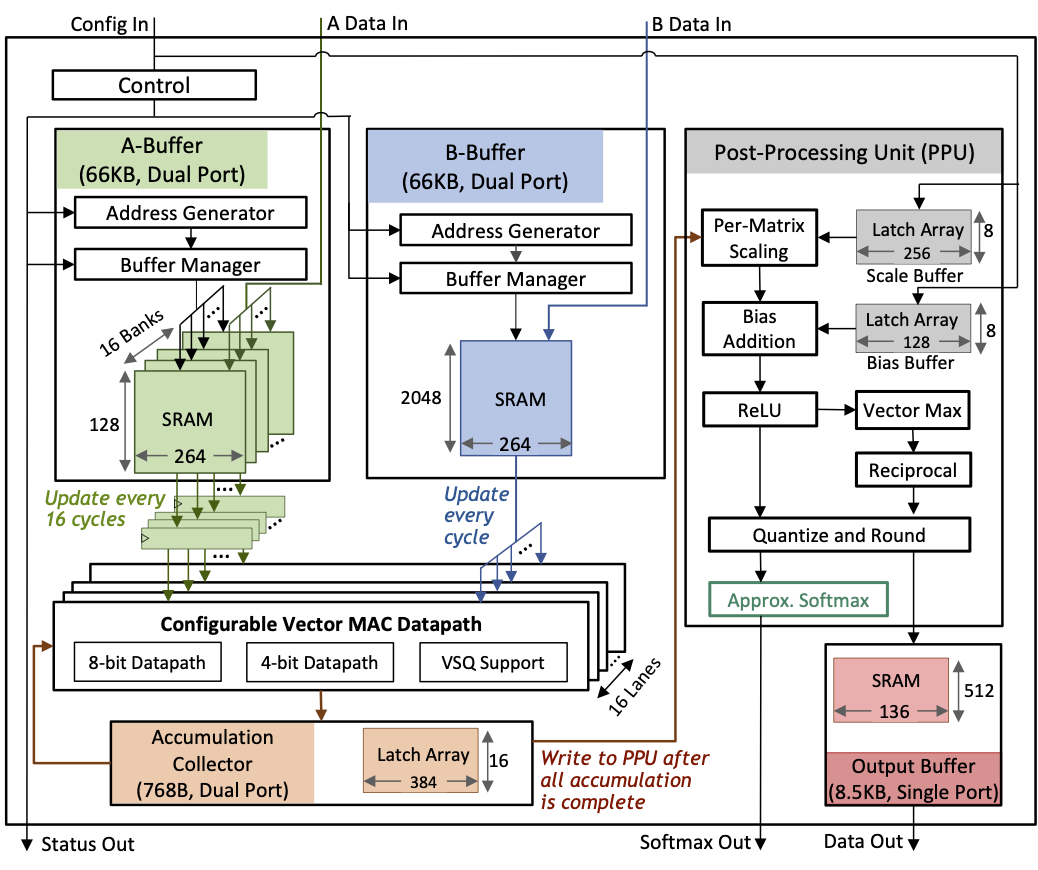
We present a deep neural network (DNN) accelerator designed for efficient execution of transformer-based DNNs, which have become ubiquitous for natural language processing tasks. DNN inference accelerators often employ specialized hardware techniques such as reduced precision to improve energy efficiency, but many of these techniques result in catastrophic accuracy loss on transformers. The proposed accelerator supports per-vector scaled quantization and approximate softmax to enable the use of 4-bit arithmetic with little accuracy loss. The 5nm prototype achieves 95.6 TOPS/W in benchmarking and 1711 inferences/s/W with only 0.7% accuracy loss on BERT, demonstrating a practical accelerator design for energy-efficient inference with transformers.
Publication Date
Uploaded Files
Copyright
This material is posted here with permission of the IEEE. Internal or personal use of this material is permitted. However, permission to reprint/republish this material for advertising or promotional purposes or for creating new collective works for resale or redistribution must be obtained from the IEEE by writing to pubs-permissions@ieee.org.