CALM: Conditional Adversarial Latent Models for Directable Virtual Characters
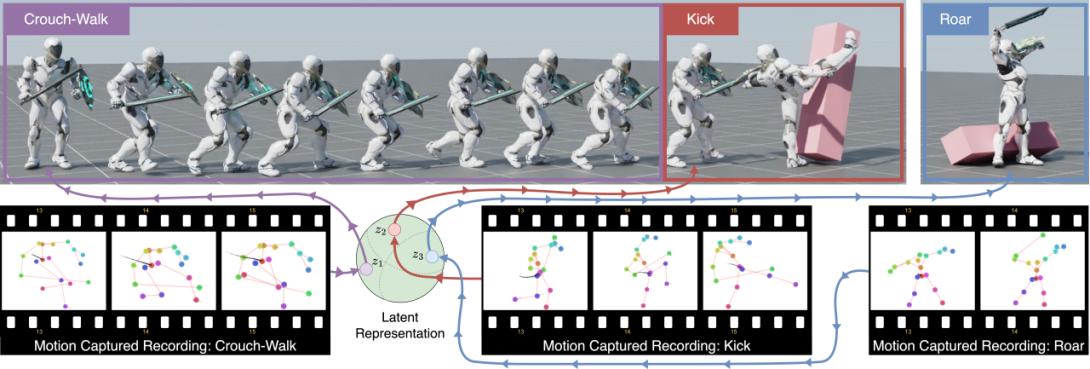
in this work, we present Conditional Adversarial Latent Models (CALM), an approach for generating diverse and directable behaviors for user-controlled interactive virtual characters. Using imitation learning, CALM learns a representation of movement that captures the complexity and diversity of human motion, and enables direct control over character movements. The approach jointly learns a control policy and a motion encoder that reconstructs key characteristics of a given motion without merely replicating it. The results show that CALM learns a semantic motion representation, enabling control over the generated motions and style-conditioning for higher-level task training. Once trained, the character can be controlled using intuitive interfaces, akin to those found in video games.