A Hybrid Generator Architecture for Controllable Face Synthesis
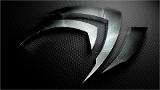
Modern data-driven image generation models often surpass traditional graphics techniques in quality. However, while traditional modeling and animation tools allow precise control over the image generation process in terms of interpretable quantities, e.g., shapes and reflectances, endowing learned models with such controls is generally difficult.
In the context of human faces, we seek a data-driven generator architecture that simultaneously retains the photorealistic quality of modern generative adversarial networks (GAN) and allows explicit, disentangled controls over head shapes, expressions, identity, background, and illumination. While our high-level goal is shared by a large body of previous work, we approach the problem with a different philosophy: we treat the problem as an unconditional synthesis task, and engineer interpretable inductive biases into the model that make it easy for the desired behavior to emerge. Concretely, our generator is a combination of learned neural networks and fixed-function blocks, such as a 3D morphable head model and texture-mapping rasterizer, and we leave it up to the training process to figure out how they should be used together. That is, we learn the distributions of the independent variables that drive the model instead of requiring that their values are known for each training image. This greatly simplifies training. Furthermore, we do not need contrastive or imitation learning for correct behavior.
We show that our design successfully encourages the generative model to make use of the interpretable internal representations in a semantically meaningful manner. This allows sampling of different aspects of the image independently, as well as precise control of the results by manipulating the internal state of the interpretable blocks within the generator. This enables, for instance, facial animation using traditional animation tools.
Publication Date
Published in
Uploaded Files
Copyright
Copyright by the Association for Computing Machinery, Inc. Permission to make digital or hard copies of part or all of this work for personal or classroom use is granted without fee provided that copies are not made or distributed for profit or commercial advantage and that copies bear this notice and the full citation on the first page. Copyrights for components of this work owned by others than ACM must be honored. Abstracting with credit is permitted. To copy otherwise, to republish, to post on servers, or to redistribute to lists, requires prior specific permission and/or a fee. Request permissions from Publications Dept, ACM Inc., fax +1 (212) 869-0481, or permissions@acm.org. The definitive version of this paper can be found at ACM's Digital Library http://www.acm.org/dl/.